Table of Contents
The human brain, with its intricate network of billions of neurons, constantly buzzes with electrical activity. This neural symphony encodes our every thought, action, and sensation. For neuroscientists and engineers working on brain-computer interfaces (BCIs), deciphering this complex neural code has been a formidable challenge. The difficulty lies not just in reading brain signals, but in isolating and interpreting specific patterns amidst the cacophony of neural activity.
In a significant leap forward, researchers at the University of Southern California (USC) have developed a new artificial intelligence algorithm that promises to revolutionize how we decode brain activity. The algorithm, named DPAD (Dissociative Prioritized Analysis of Dynamics), offers a novel approach to separating and analyzing specific neural patterns from the complex mix of brain signals.
Maryam Shanechi, the Sawchuk Chair in Electrical and Computer Engineering and founding director of the USC Center for Neurotechnology, led the team that developed this groundbreaking technology. Their work, recently published in the journal Nature Neuroscience, represents a significant advancement in the field of neural decoding and holds promise for enhancing the capabilities of brain-computer interfaces.
The Complexity of Brain Activity
To appreciate the significance of the DPAD algorithm, it’s crucial to understand the intricate nature of brain activity. At any given moment, our brains are engaged in multiple processes simultaneously. For instance, as you read this article, your brain is not only processing the visual information of the text but also controlling your posture, regulating your breathing, and potentially thinking about your plans for the day.
Each of these activities generates its own pattern of neural firing, creating a complex tapestry of brain activity. These patterns overlap and interact, making it extremely challenging to isolate the neural signals associated with a specific behavior or thought process. In the words of Shanechi, “All these different behaviors, such as arm movements, speech and different internal states such as hunger, are simultaneously encoded in your brain. This simultaneous encoding gives rise to very complex and mixed-up patterns in the brain’s electrical activity.”
This complexity poses significant challenges for brain-computer interfaces. BCIs aim to translate brain signals into commands for external devices, potentially allowing paralyzed individuals to control prosthetic limbs or communication devices through thought alone. However, the ability to accurately interpret these commands depends on isolating the relevant neural signals from the background noise of ongoing brain activity.
Traditional decoding methods have struggled with this task, often failing to distinguish between intentional commands and unrelated brain activity. This limitation has hindered the development of more sophisticated and reliable BCIs, constraining their potential applications in clinical and assistive technologies.
DPAD: A New Approach to Neural Decoding
The DPAD algorithm represents a paradigm shift in how we approach neural decoding. At its core, the algorithm employs a deep neural network with a unique training strategy. As Omid Sani, a research associate in Shanechi’s lab and former Ph.D. student, explains, “A key element in the AI algorithm is to first look for brain patterns that are related to the behavior of interest and learn these patterns with priority during training of a deep neural network.”
This prioritized learning approach allows DPAD to effectively isolate behavior-related patterns from the complex mix of neural activity. Once these primary patterns are identified, the algorithm then learns to account for remaining patterns, ensuring they don’t interfere with or mask the signals of interest.
The flexibility of neural networks in the algorithm’s design allows it to describe a wide range of brain patterns, making it adaptable to various types of neural activity and potential applications.
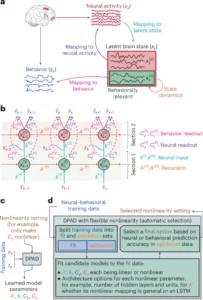
Source: USC
Implications for Brain-Computer Interfaces
The development of DPAD holds significant promise for advancing brain-computer interfaces. By more accurately decoding movement intentions from brain activity, this technology could greatly enhance the functionality and responsiveness of BCIs.
For individuals with paralysis, this could translate to more intuitive control over prosthetic limbs or communication devices. The improved accuracy in decoding could allow for finer motor control, potentially enabling more complex movements and interactions with the environment.
Moreover, the algorithm’s ability to dissociate specific brain patterns from background neural activity could lead to BCIs that are more robust in real-world settings, where users are constantly processing multiple stimuli and engaged in various cognitive tasks.
Beyond Movement: Future Applications in Mental Health
While the initial focus of DPAD has been on decoding movement-related brain patterns, its potential applications extend far beyond motor control. Shanechi and her team are exploring the possibility of using this technology to decode mental states such as pain or mood.
This capability could have profound implications for mental health treatment. By accurately tracking a patient’s symptom states, clinicians could gain valuable insights into the progression of mental health conditions and the effectiveness of treatments. Shanechi envisions a future where this technology could “lead to brain-computer interfaces not only for movement disorders and paralysis, but also for mental health conditions.”
The ability to objectively measure and track mental states could revolutionize how we approach personalized mental health care, allowing for more precise tailoring of therapies to individual patient needs.
The Broader Impact on Neuroscience and AI
The development of DPAD opens up new avenues for understanding the brain itself. By providing a more nuanced way of analyzing neural activity, this algorithm could help neuroscientists discover previously unrecognized brain patterns or refine our understanding of known neural processes.
In the broader context of AI and healthcare, DPAD exemplifies the potential for machine learning to tackle complex biological problems. It demonstrates how AI can be leveraged not just to process existing data, but to uncover new insights and approaches in scientific research.